Avoiding Technology Tarpits: Ontology and Taxonomy
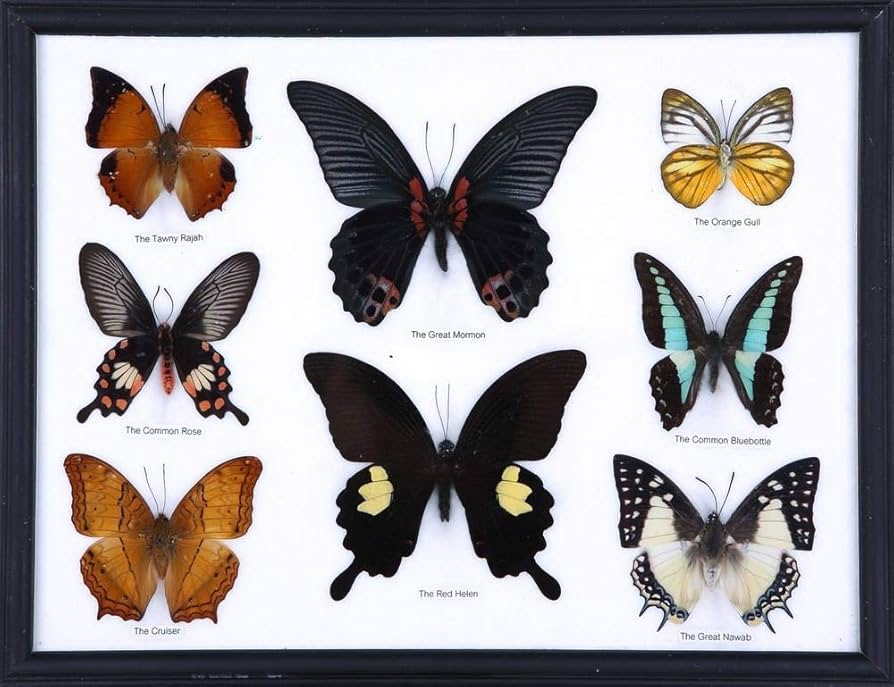
Avoid, avoid, avoid starting a project by modeling your data in an ORM. Why? Because the temptation in data modeling is to model the "thing" perfectly instead of prioritizing your model by utility within your domain. I have seen countless projects die a slow unsatisfying death because their attempt to capture everything devolved into never-ending Doneness Creep – a subspecies of feature creep where you convince yourself that your product is never ready enough to release. Usually this is accompanied by a Design By Committee flaw.
Stated simply, the Taxonomy and Ontology tarpits are:
- The taxonomy tarpit is when you have a classifier or category system, and you keep finding things that don't fit neatly into the category, or where the hierarchy is never quite sufficient to navigate your collection.
- The ontology tarpit is when you continuously add to the attributes and validation of your model regardless of those things necessity. Often there are use-cases for these, but there's no effective discriminator between "necessary" and "nice to have" and "can wait till later"
It's difficult to provide examples of these, because the products that devolve into them are almost always DOA. You've run into them in your career though if you've been in the industry long enough.
Take a person. A "profile" might be the standard attributes offered up in Cognito. But what is a person, really? What attributes provide a complete enough picture that you'd never need more?
Without the discriminator of the domain model you can get lost in any manner of rabbit hole. You could boldly assume that standard attributes are enough when they aren't. You could have useless attributes that you add "just in case" they're ever needed. You can spend days figuring out how to store every variant of phone numbers and email addresses and how to validate them when validation isn't actually required.
Or you could not do that. Instead you could say:
- What queries use profile data, and what attributes do they need to function performantly and reliably? (queries)
- How is data expected to get into the system and be modified? What are the mutations and commands that drive your system's behavior? (commands)
- Which attributes drive system or human behavior and therefore need validation on entry? (behavior)
- How many people and different use-cases do I expect will pile onto this product as soon as I release it? (roadmap and scale)
Now you have questions that tell you what data is necessary, what you can leave out and what can wait till later. "Domain Modeling" has taken on a whole meaning of its own and there are plenty of people who will sell you expensive tools and frameworks to do it. These are only needed as they're helpful though. What you really need to do is understand what's being asked of your software, and here some simple exercises and market research are pretty sufficient.
Question 1: Queries
This asks, "when people or other systems get data out of this system, what do they need and how do they expect to access it?"
For people, this is often some form of decision support or compliance activity. They typically need to be able to get a high level listing for the purpose of browsing what data is available. For processes, you often have more "fixed" than exploratory queries, and you're typically optimizing for system load, throughput, and latency.
Think about the people or UIs accessing these queries. Depending on the number of items, they will need pagination and different sort methods. Also depending on the number of items, they'll need search. That is, if you're searching people by name, you need to be able to account for misspellings and therefore want a trigram index or a phonetic attribute for names. Are you fully utilizing unicode or do you need directional writing support out of the gate (lucky you)? How will the search be parameterized?
Finally, performance. A query that powers a once-a-day process running in the background can take an hour. Who cares, as long as you can time it and you can constrain the resources it takes up on the system? A query that powers a UI needs to return in 90ms or so, and if it fails for some reason that reason needs to be clear since a simple retry won't likely do and you need to give the user some useful action.
Understanding what queries are going to be executed, by whom, and with what expectations of responsiveness tells you what you need to store and how you need to store it.
Continuing our example: we go figure out who our likely first users are who need significant amounts of profile data. Let's say this is a system for managing the people for our regional coffee roaster and shop chain. We have:
- On the order of 25,000 profiles that one person might have access to. We hope that number will grow to the millions. All our profiles and users are Canadian, so we have to support French characters and phonetic search in addition to English.
- A process that runs weekly to send out newsletters to email addresses.
- A process that runs monthly to send out mailers to people.
- A process that runs at will to send a pound of a person's preferred bean, grind, and roast to the address they gave.
- A UI where I can search profiles by territory, interest group (coffee, tea, accessories), and by name, and as an admin view and modify data.
- A login interface for anyone in that list of profiles where they can see and correct their data as well as sign up for a coffee or tea subscription.
Those requirements tell me a lot more about how profiles need to be structured in the system and which attributes are essential than my initial stab at "Amazon has standard attributes and is a pretty big company, so they're probably right."
Question 2: Commands
Is the data coming from another system? Is it being uploaded in bulk via CSV, JSON, Excel? Who can modify it? You'll figure out validation and such in the next question, but for this one you really want again to model utility patterns and performance.
Maybe there is a bulk upload interface, but the CSV files aren't going to have people's coffee preferences or buying habits in them. So you know that you have to support bulk import, but some attributes will be blank. Now you know that something that you might have required can't be.
And again, there's the question of performance. If a person is updating their profile, the update can take a little longer than the load, but not much. If the guts of your system make it so that certain updates take a bit, those are going to have to be asynchronous.
Question 3: Behavior
Reiterating from question 1 the processes our system drives and UI it affords in our fictional coffee chain, we have:
- A process that runs weekly to send out newsletters to email addresses.
- A process that runs monthly to send out mailers to people.
- A process that runs at will to send a pound of a person's preferred bean, grind, and roast to the address they gave.
- A UI where I can search profiles by territory, interest group (coffee, tea, accessories), and by name, and as an admin view and modify data.
- A login interface for anyone in that list of profiles where they can see and correct their data as well as sign up for a coffee or tea subscription.
Given the above, we can say pretty confidently that our system needs, as attributes:
- Preferred and given name
- Phonetic searchable indexed attributes for the above (likely a trigram index).
- Market region (e.g. Toronto, Windsor, Hamilton, Ottowa, Montreal)
- Opt-in or opt-out attributes to let me know whether to check subscription tables.
- Interest tags (coffee, tea, etc)
- Physical address (validated)
- Billing address (validated, but maybe by my payment processor)
- Email address (validated)
Question 4: Roadmap and Scale
This is where you get to the M of the MVP. An MVP isn't simply a half-finished product that serves a need just enough better than the solution people are already using to make them convert. It often is that, but it doesn't have to be. If you're really clear about applying the razor of necessity to all parts of your product, you can use the saved time to put the effort in to make your Minimum Viable Product a joy to use.
Given the scale we expect at the beginning – 25,000 profiles – and the use cases, we don't expect there to be a lot of traffic up front. Maybe a few hundred daily active users. At that scale the only index that is actually necessary is the trigram index because calculating string distance or phonetic matches is expensive work to waste. It could even be in-memory if you don't want to use OpenSearch for some reason and you really just enjoy the pain of writing search from scratch for a few dozen searches a day, but it does need to be there.
We know we're starting with 25,000 customers, but we'd like to eventually scale to a million plus. It might be a few years though. Also, within a few months of release we want to be able to keep people's favorite drinks so they can place an order from their Apple Watch when they walk into one of our shops.
It's good to know what scale you're going to get to, and it's good to have some other use-cases that you know are coming down the pipeline. But you can't support all use-cases or scale out of the gate. If you try to capture all of them, you're right back at the tarpits we're talking about. Instead, you have to build your system to evolve. You'll need to be able to add attributes down the road, add more indexes, validation, or specialized data stores, and recognize that attributes are sometimes complex in the sense that they comprise two or more linked fields.
Wrapping it up
Taxonomy and Ontology traps are some of the most common ways for projects to devolve and fail. You will eventually run into one trying to form if you work long enough. Asking the right questions up front, rather than starting by modeling the objects and their attributes is the right way to approach domain modeling to keep this from happening.
Model external interactions (commands and queries), behavior, initial scale and roadmap, and design your system to be evolvable along those lines, and you will avoid the temptation to figure out everything before you can get started.